Artificial Intelligence has become a game-changer in logistics, optimizing critical operations such as parcel sorting and piece picking. These tasks, which once required significant manual labor, are now being automated with AI’s ability to analyze, decide, and act in real-time.
Table of contents
- 3D Camera+AI: How Does It Work?
- Zivid’s Role in Enhancing AI Interpretation
- Logistics Automation Depends on AI and 3D
- Fizyr – Parcel Sorting
- Siemens – Piece Picking
- Maximize the Effectiveness of AI by Choosing the Right 3D Camera
However, the success of AI in these complex environments hinges on one key factor: the quality of the data it processes. This is where 3D vision technology enters the equation, providing AI with the precise visual data necessary to interpret and interact with the physical world.
3D Camera+AI - How Does It Work?
In the context of logistics, where tasks like parcel sorting and piece picking involve dealing with thousands of items of different, hard-to-predict shapes, sizes, and materials, 3D vision provides AI with the rich, precise data it needs to function effectively.
Capturing the Scene: Point Clouds
A point cloud consists of millions of individual data points, each representing the precise X, Y, and Z coordinates of a point in the scene. Industrial 3D cameras generate point clouds that capture objects' spatial dimensions regardless of surface properties, such as texture, reflectivity, and color.
Note: You can downsample and limit the region of interest using Zivid SDK and GUI (Zivid Studio), so the processing takes even less time.
Data utilization: The power of unified vision data
In logistics parcel handling and piece-picking, there are different approaches to object identification and segmentation. Some approaches use high-resolution 2D to identify objects and 3D point clouds for pose estimation and picking strategy. Some also choose to blend 2D and 3D data. Yet another approach is to use normal data to identify the surface, edges, and positional angle of the object.
Different strategies will suit particular use cases. What is highly beneficial is a 3D camera that can deliver all data (XYZ, 2D, normals, depth map) in a coherent pixel-wise manner, such as Zivid 2+. This means that all this data is available and correlated for each point, making it far simpler and more reliable to employ different approaches using a single dataset from a single device.
Feeding Data into AI Vision Software
Once the 3D camera captures a point cloud, this data is fed into AI vision software. The software’s first task is to interpret all or parts of this dense cloud of data to make sense of the scene. This typically involves several steps:
1. Point Cloud Preprocessing and Object Segmentation
The software begins by filtering out noise and irrelevant data points. The software then segments the point cloud into distinct regions or objects. This involves grouping points that are close together and likely to belong to the same object. This step is crucial for applications like piece picking, where the AI needs to distinguish between different items in a cluttered environment.
2. Object Detection and Classification
After segmentation, the AI analyzes the shapes and features of the segmented regions to identify them. This could involve comparing the shapes against a database of known objects or using deep learning models to classify objects based on their 3D shapes and textures. For example, in a parcel sorting center, the AI might classify different packages based on size, shape, and material, determining the best way to handle each item.
3. Pose Estimation
Once an object is identified, the software determines its position and orientation in space, known as pose estimation. This is where the high accuracy of Zivid’s cameras becomes critical. Accurate pose estimation allows the AI to determine the exact location and angle of the object, which is essential for guiding a robot to pick or manipulate the object correctly and avoid under or over-shooting during the picking operation.
4. Decision-Making and Action Triggering
The AI can make informed decisions with all the information gathered—object identity, position, orientation, and other relevant data. For instance, in a piece-picking scenario, the AI might decide the optimal approach angle and grip force for a robotic arm to pick up an item. The AI then triggers the appropriate actions, such as instructing a robot to move to a specific location, picking the object with a suction gripper, or placing it in the bin.
Zivid’s Role in Enhancing AI Interpretation
Zivid’s 3D cameras are designed to produce point clouds that are of the highest quality and completeness with minimal noise and distortion. As with any AI system, its performance is usually dependent on the quality of the dataset it is using during inference. The cameras’ ability to capture fine details, such as the edges of transparent or shiny objects, ensures that the AI can access the best possible data, enabling it to make precise and reliable decisions.
SIMATIC Pick AI using Zivid 2+ camera, deployed at Mecalux
Moreover, Zivid’s wide field of view and high spatial resolution mean that even large scenes can be captured in a single frame, with no loss of detail. This is crucial for applications where the AI needs to assess an entire scene quickly, such as when dealing with multiple items in a bin-picking scenario.
Logistics Automation Depends on AI and 3D
Unlike in manufacturing, where workpieces are often identical and predictable, logistics and eCommerce environments deal with thousands of distinct SKUs (stock keeping units) and many of these items can differ significantly due to packaging variations. Those items need to be picked, sorted, and packaged. For this process to be automated, robots must be faster and more precise than humans. Robots need brains and eyes.
Since launching our Zivid 2+ family, we have worked with multiple logistics solution providers that had amazing AI solutions but struggled to find the right 3D camera to complement their software. There just wasn’t a camera that could provide data on wrapped items, transparent or shiny consumer goods.
With our technology, you can clearly see transparent objects, wrapped items, and shiny materials
Our Omni Engine changed the game and introduced revolutionary vision technology, enabling AI with enough data to interpret. Let's check out cases of Zivid cameras enabling AI for logistics automation.
Fizyr – Parcel Sorting
Packages in parcel sorting vary widely in size, shape, and material, presenting a significant challenge for automated systems. Fizyr, a leader in deep learning for logistics, recognized early on that traditional methods would not suffice. They needed a system to learn and adapt quickly to handle an average warehouse's enormous variability of parcels.
The demo of this solution was showcased at the Automate Show 2024
Fizyr’s software, in combination with Zivid’s high-quality 3D point clouds, delivers exactly that. The Zivid 3D cameras provide the precise data that Fizyr’s AI uses to detect and segment objects, identify optimal pick poses, and easily navigate complex environments.
System integrator AWL recognized this partnership by integrating it into their Robotic Singulator Cell. This solution enables accurate decision-making in under 300 milliseconds, allowing for faster and more reliable parcel sorting. It sets new standards for speed, accuracy, and reliability in warehouse automation.
Siemens – Piece Picking
Siemens has developed the SIMATIC Robot Pick AI, a deep learning-based vision software that excels in such environments. By integrating Zivid’s 3D cameras, Siemens enhances its system’s ability to make real-time decisions on pick points without relying on pre-existing CAD models. The Zivid cameras provide the high-resolution, accurate point clouds that Siemens’ AI uses to understand and manipulate various items, ensuring precise and reliable performance.
In a recent demo, Siemens showcased this solution using Zivid’s cameras and a UR 20 robot arm. The demonstration highlighted how the integration of a highly accurate 3D camera enhances the AI’s capability to perform complex piece-picking tasks, making it an ideal choice for logistics operations.
You can watch the whole webinar about this solution for free!
Maximize the Effectiveness of AI by Choosing the Right 3D Camera
The integration of AI and 3D vision is not just enhancing current logistics operations—it’s paving the way for future advancements. The demand for precise, high-quality visual data will only grow as AI continues to evolve. Companies that invest in advanced 3D vision technology today will be well-positioned to capitalize on the next wave of innovation in logistics.
Zivid’s 3D cameras are at the forefront of this transformation, providing the critical visual data that AI systems need to excel in logistics applications. By combining the strengths of AI and 3D vision, logistics operations can achieve new levels of efficiency, accuracy, and reliability.
- Accuracy and Precision: Zivid 2+ cameras deliver exceptional accuracy, with dimension trueness errors of less than 0.2% for the M60 and less than 0.4% for the L110, ensuring sub-millimeter precision in all conditions.
- High-Quality Data: Zivid cameras are designed to produce high coverage, low distortion, and low noise, generating the quality point clouds that AI systems depend on.
- Wide Field of View: Despite a 60-degree opening angle, Zivid cameras maintain high spatial resolution with 5Mpx, allowing robots to capture entire scenes in rich detail, critical for applications like piece picking.
Want to know more? Contact our sales representative!
You May Also Like
These Related Stories
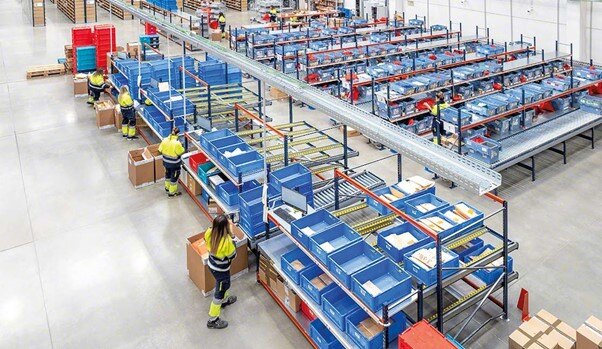
Why Low-End 3D is Hurting your Warehouse Piece-Picking Entitlement
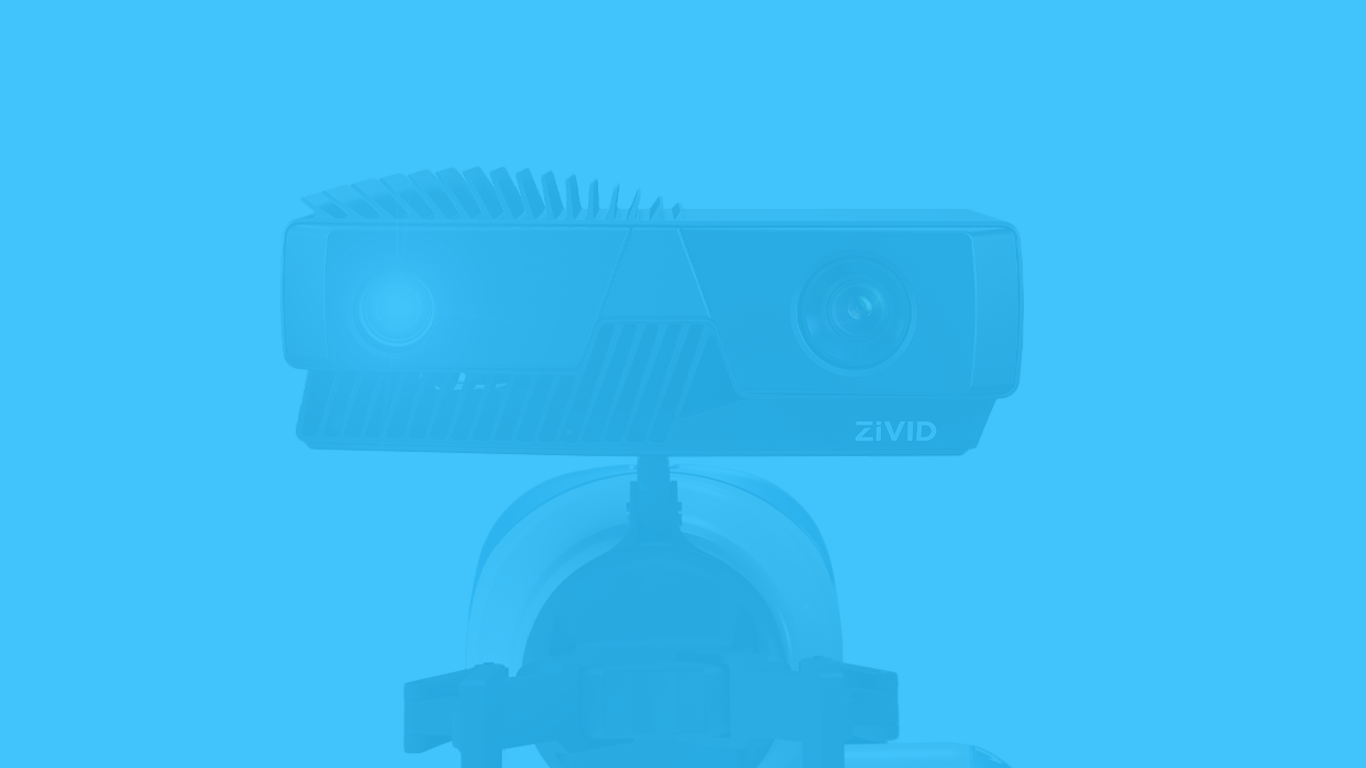
A beginner's guide to 3D machine vision cameras
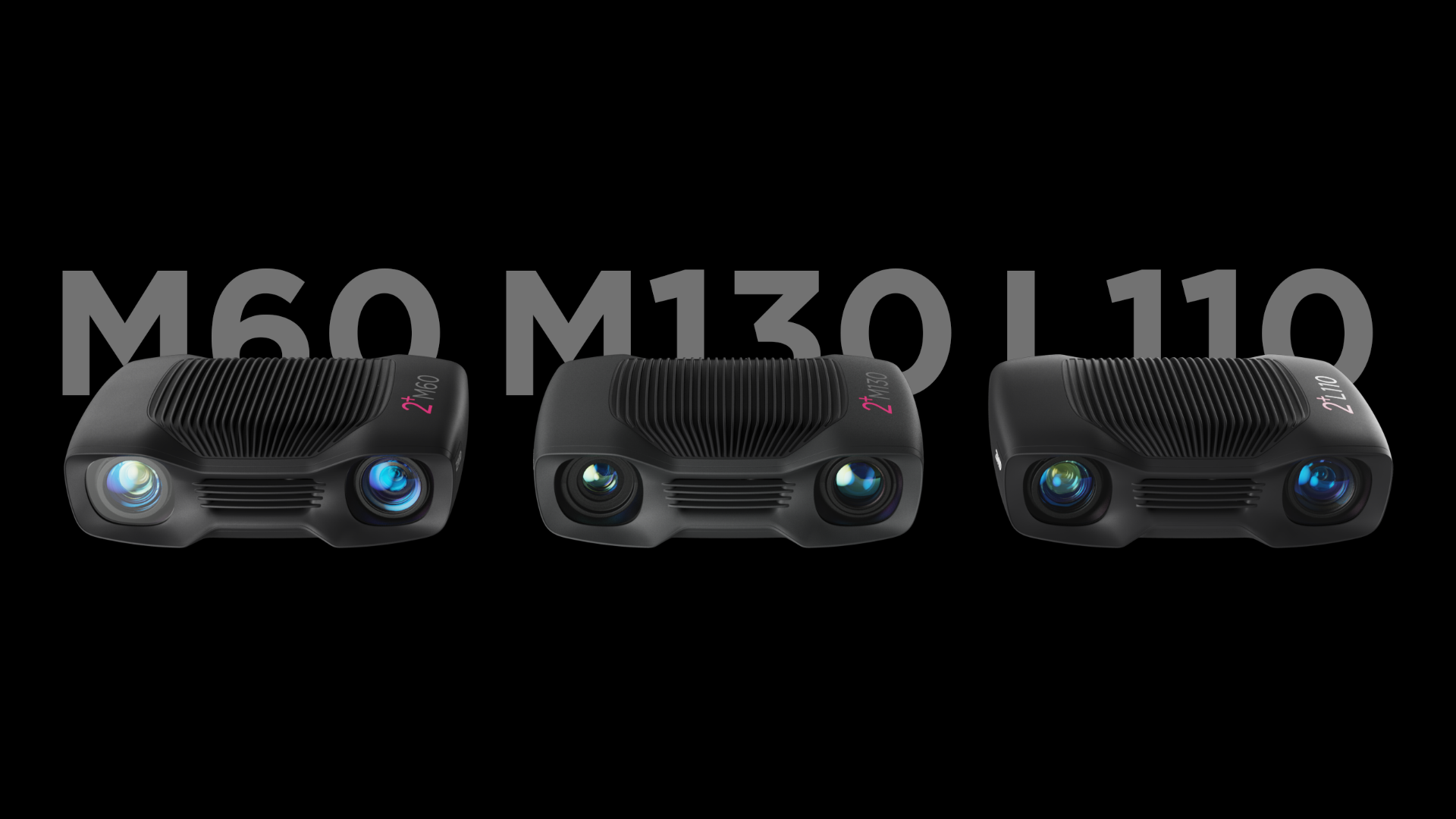