Why You Need to Capture in Both 3D and 2D for Order Picking
Integrating both 2D and 3D data capture is crucial for piece-picking (or “order picking”) applications. With piece-picking and order fulfillment, you are often dealing with thousands upon thousands of items of all shapes and sizes. When you have this level of variety, it is critical to get all the information you can to tell items apart.
What do you do when you have multiple types of the same face masks, and you need to distinguish them? What about types of chips? With 3D data alone, you won’t be able to tell them apart. This is just part of why order picking can not rely on 3D information alone, and 2D is critical to accurate picking.
Table of contents
- Advantages of 2D+3D Imaging
1. The importance of 2D data
2. The power of 3D imaging
3. Limitations of CAD matching
4. Enhanced detection and picking
5. Improved flexibility and control
6. Optimized processing speed - Case studies with Zivid
- Conclusion
The Advantages of 2D+3D Imaging
While 2D imaging provides critical color and texture information, 3D imaging offers depth and spatial details, making the combination of these technologies a powerful tool in automated piece-picking applications.
The Importance of 2D Data
2D imaging is a staple in machine vision due to its high resolution and ability to capture detailed color information. Really, this is where all machine vision started. Most of the identification of consumer goods is still done with the 2D data. It is particularly useful for identifying and differentiating between objects based on their visual appearance, especially with similarly shaped packaging. In an order-picking context, 2D images can be used to read labels, recognize logos, and detect surface defects.
Often, machine vision algorithms and AI use 2D data to identify items and differentiate them from their neighbors. When dealing with a large variety of items and a changing inventory, these identification methods are needed since CAD matching with 3D might not be possible.
The Power of 3D Imaging
Once you know where your pickable item is in 2D, 3D imaging captures the depth and spatial characteristics of objects. This is essential for determining the exact position, orientation, and volume of items. In cluttered environments, 3D data helps accurately identify and isolate objects, reducing the chances of picking errors by pick-and-place robots. The slides below explain more about picking errors:
Having high-quality and accurate 3D data also helps decrease the risk of damaging the items you want to pick. 3D data can come in a whole quality scale, from lumpy surfaces to clean, sharp edges and details. When you use higher-quality 3D data, you can pick with surety. This can allow for faster robot movements, grippers needing less compliance, and less downtime for the order fulfillment solution.
Compare point cloud quality from different 3D cameras on the market →
Limitations of CAD Matching
Relying solely on CAD matching, which uses only 3D data, is not a viable option for order picking due to its inability to handle the complex and varied nature of real-world objects effectively. CAD models are typically precise and idealized representations that may not account for the inconsistencies and variations found in actual products, such as differences in packaging, wear, or damage. Moreover, CAD matching lacks the ability to recognize surface details and colors, which are critical for identifying and differentiating between similar items in a diverse inventory.
Another limitation of CAD matching is picking items that have deformable packaging, such as plastic bags. A key example here would be the clothing industry. Often, clothes are shipped in thin plastic bags that are easy to bend, fold, and crumple. A CAD algorithm would not be possible here.
The absence of 2D information can lead to increased picking errors and inefficiencies, especially in environments with high SKU variability and complex object geometries.
Enhanced Detection and Picking
The combination of 2D and 3D imaging leverages the strengths of both technologies. While 2D imaging excels in capturing detailed visual information, 3D imaging ensures precise localization and depth data. This synergy significantly enhances the detection accuracy and reliability of e-commerce picking systems, ensuring that items are correctly identified and handled.
Download free eBook: Order Fulfillment and Intra-Logistics with 3D Vision →
Improved Flexibility and Control
Separate 2D captures allow for better control over camera settings, such as resolution, focus, and lighting, optimizing the image quality for the scene. This flexibility is crucial in environments with varying lighting conditions or where high-resolution color data is required for object differentiation. Often, systems can rely on having lower-quality 3D data to pair with high-quality 2D data.
For an example of how to capture both 2D and 3D independently with Zivid, check out the Python sample.
Optimized Processing Speed
By strategically using 2D data from 3D captures or performing separate 2D captures, it is possible to optimize the processing speed. Disabling RGB in 3D captures when high-quality 2D images are needed can save processing time, leading to faster cycle times and more efficient operations. In some cases, systems might rely on using the 2D capture only until the object of interest enters the scene. With this information, it is possible to only take the slower 3D capture when necessary.
Case studies with Zivid
3D vision cameras, such as those provided by Zivid, have proven effective in various industrial applications. These cameras use structured light to create detailed 3D point clouds, which can be enhanced with color data from 2D imaging for precise object classification and sorting.
Until recently, most 3D cameras only did 3D data and a gray scale image for 2D. Because of this, automated cells needed a second camera that would provide color. This camera then had to be calibrated to match the 3D camera so you could correlate all the pixels correctly. This process can be prone to errors and often requires a specific ridge jig between the two cameras. This increases the cost by not only one camera but also hardware infrastructure. Many times, you will also need external lighting to get proper color data for the external camera. At this point, you are not building a system with just one extra camera but a connection jig, more lighting, hardware for lighting, and a large time investment with extra calibration.
Zivid has 2D and 3D in the same camera and with good quality color 2D data as well. With unified 3D+2D, our customers only need 1 camera to get both data types and do not need secondary calibration.
Check out some examples of customers who are using Zivid cameras to complete complex piece-picking scenarios:
Conclusion
Incorporating both 2D and 3D imaging in order-picking systems provides a robust solution to the challenges of automated piece picking. The combination ensures high accuracy, flexibility, and efficiency, making it an indispensable strategy in modern warehouse automation. By understanding and leveraging the unique advantages of both 2D and 3D captures, businesses can significantly enhance their order-picking processes.
For more detailed insights and technical guidelines, refer to the full article on the Zivid Knowledge Base.
You May Also Like
These Related Stories
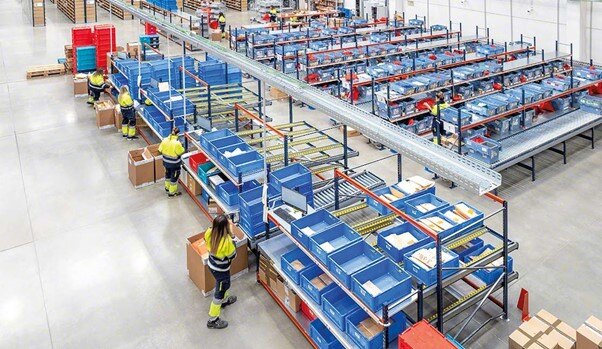
Why Low-End 3D is Hurting your Warehouse Piece-Picking Entitlement
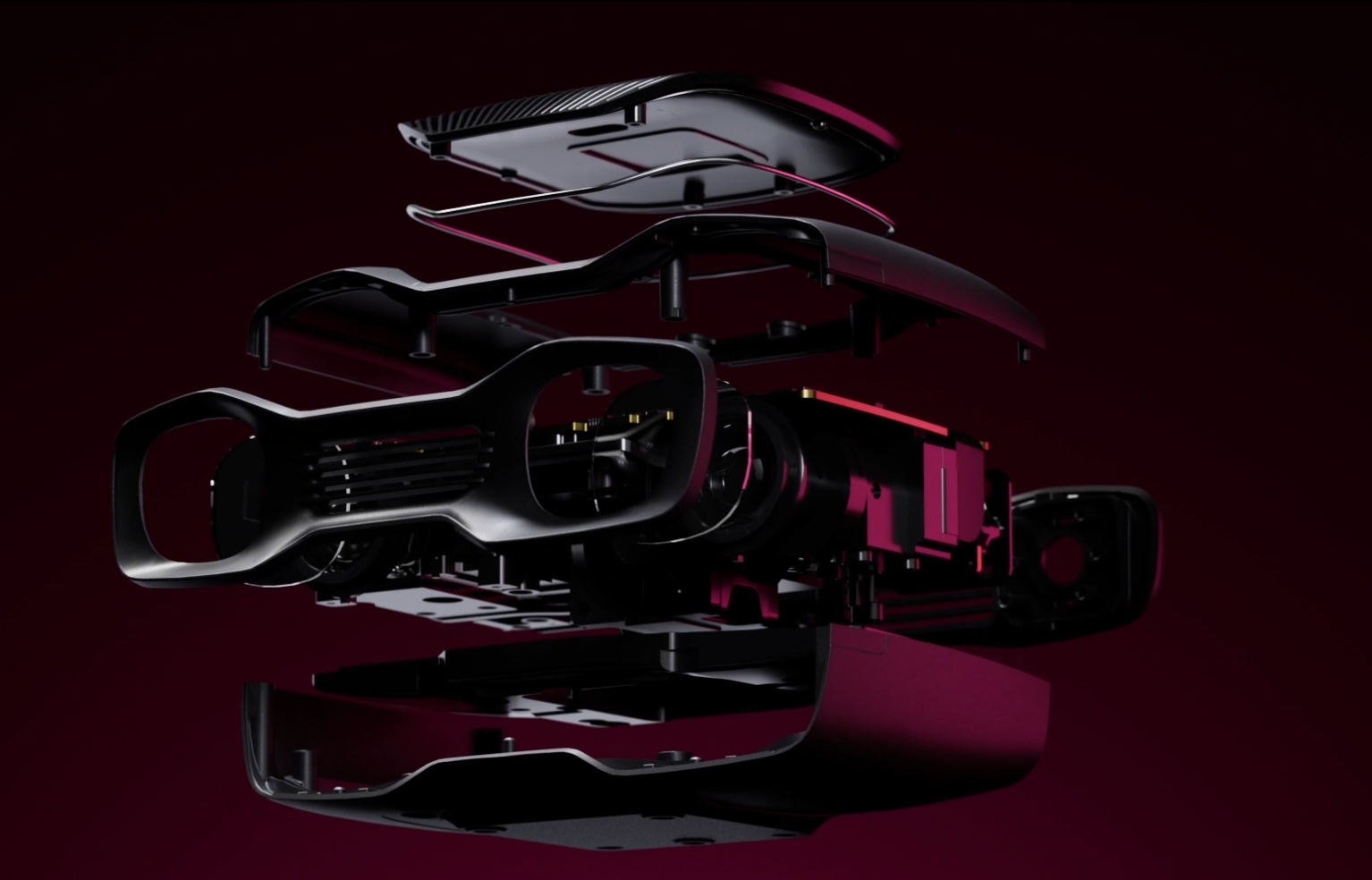
Zivid 2+ R-series: 3x Faster and Better Vision for your Robot
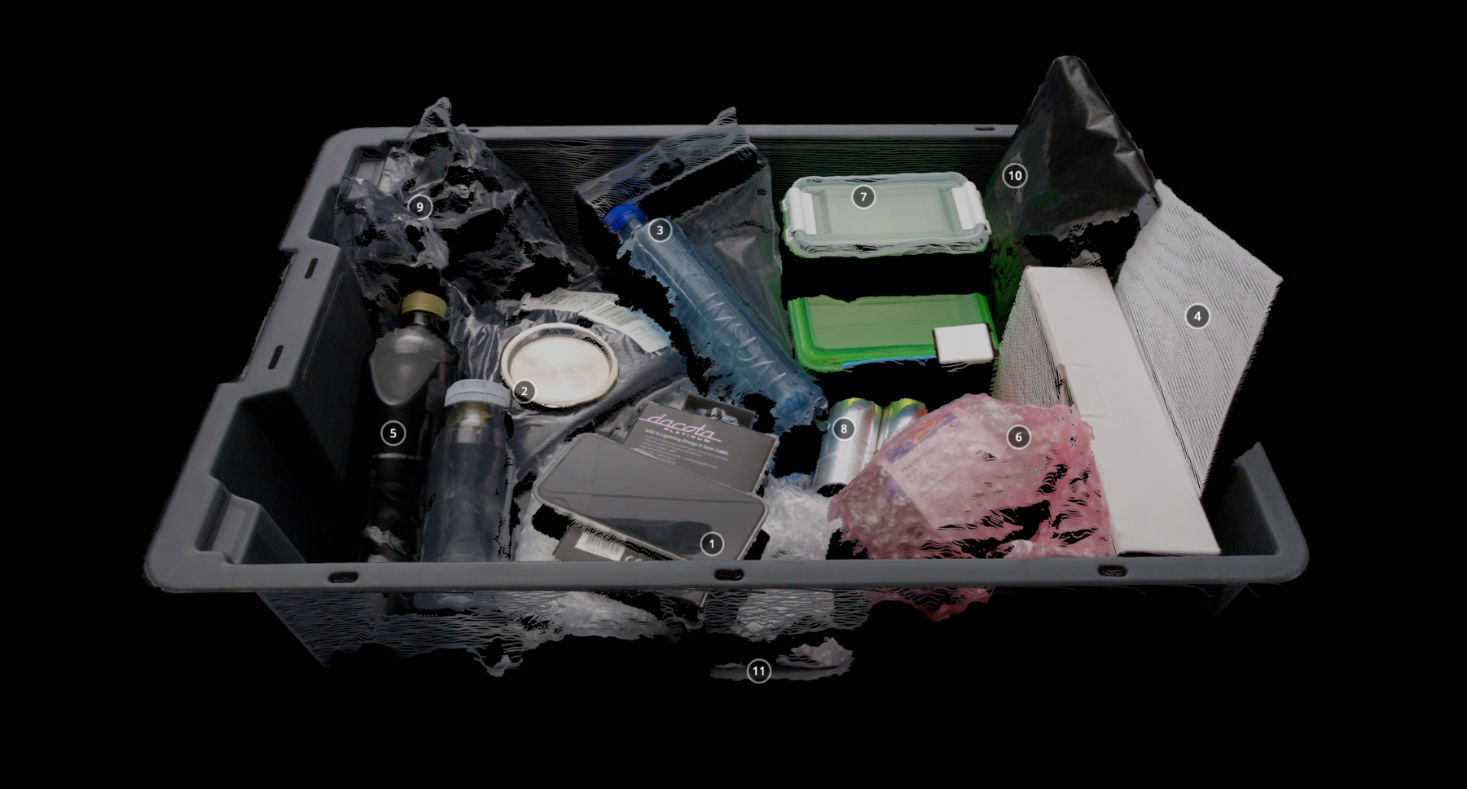