Impact of 3D vision in vision-guided robotic applications
This blog article is based on the webinar, Impact of 3D vision in vision-guided robotic applications, hosted by our European distribution partner, Stemmer Imaging. In this webinar, you will learn how high-quality point clouds from Zivid 3D cameras can improve the functionality, reliability, and efficiency of vision-guided robotic applications.
Agenda
- The 3D vision camera – What role does it play?
- See with Confidence – Benchmarking of 3D point clouds from different 3D vision sensors.
- Pick with Confidence – Why trueness matters in 3D imaging?
- On-arm versability – Unleashing the potential of on-arm mounting.
- Pick and place robotic applications – Real-world examples
- Live demo - Zivid Two 3D camera
- Q&As
1. The 3D vision camera – What role does it play?
What role does 3D vision play in today's pick and place robotic applications? We know that the 3D vision system plays an essential role in each of the stages; Detect, Pick, and Place.
During 'Detect', 3D cameras enable your robot to see and scan a target object or a particular scene. The output provided by a 3D vision camera is a point cloud. The 3D point cloud quality must be good enough for the software to distinguish, recognize and match parts clearly to perform steps like picking and placing.
With years of in-field experience, we have understood and realized that the 3D vision system majorly affects the ‘’Picking’’ part of the automated pick and place application. Picking an object becomes more reliable when you have true-to-reality data. It means that your 3D vision system must provide a correct representation of object size, rotation, and absolute position, as true to reality as possible, to the robot coordinate system. Higher quality of point-cloud reduces the risks of mis-picks and enables the robot gripper to grip and pick a part from an exact point.
The final stage, that is, ‘’Placing’’, also requires high-quality 3D vision. Not all applications require a careful drop-off, but being able to place a part in a specific location without colliding or damaging the part is crucial in many of today's vision-guided pick and place applications.
Overall, 3D vision plays a crucial role in each step of the vision-guided robotic applications.
Let’s take some examples of typical pick and place robotic applications. Here are three common applications we see in the automation market.
The first application is piece picking for automated order fulfillment in e-commerce, logistics, and smart warehouses. The ideal solution is to cover and detect a full range of stock-keeping units (SKU) available in such an environment. If your vision system cannot detect all the parts or get close to full coverage of all SKUs available, you won’t succeed in piece picking. This is one of the main challenges in piece picking today. Learn more about piece picking challenges.
The next application is bin-picking which is widely known and used in industrial automation applications. Your robot is required to pick a target object from one bin and place it in other bins, a tote, or any other desired location. A key challenge with bin-picking is to empty the bin completely. It is hard for the robot to pick the parts when they are randomly arranged and piled on top of each other. Additionally, the parts close to the bin walls can be challenging to pick due to occlusion errors, which need to be considered before choosing a vision system. Learn more about bin-picking challenges.
The last and emerging application in the automotive and manufacturing industry is machine tending. A robot needs to load and unload parts from one location into machines for further processing in machine tending. Some machine tending scenarios require inserting or fitting industrial objects like cylinders into a feeding hole or arrange them in a fixture in a uniform way. This is not easy to achieve without a highly accurate vision system due to the tight tolerances and high accuracy required for picking and placing tasks. Learn more about machine tending challenges.
2. See with Confidence – Benchmarking of 3D point clouds from different 3D vision sensors
In this chapter, you will see how the quality of 3D data impacts applications in real-world scenarios. We’ll look at several 3D point cloud examples from leading 3D vision systems in the market.
First, we have a 3D point cloud of consumer goods that are randomly placed in an industrial bin. The bin is captured with a low-end stereo camera. This is a popular and relatively cheap 3D vision solution in the market, and it provides the result given below in approximately 66 ms.
You can see a tube and some boxes but you cannot see too many details in the 3D data. Look at the middle part, and you will notice that everything is merged, making it hard to differentiate the items.
Let’s scan the same bin with a high-end stereo camera, another popular 3D vision method in the market.
This time it took 400 ms, which is slower than the previous example. The quality of the 3D data has improved. The tube's shape is more apparent, and you can read the text on the box at the bottom left.
But what happens to the items highlighted in the circle? If I asked you to tell me what the objects are in this part, would you be able to do it?
Given that we have a human brain, probably the best AI available, and human eyes, the best vision system possible, it is still difficult to differentiate the parts. The same goes for picking robots. If your robot system cannot recognize the parts and boundaries, it cannot pick them successfully.
This is where Zivid comes into play. See what happens when you capture the same scene with our Zivid 2 3D camera.
The parts that were not recognizable are now very visible. The 3D data is sharper and more understandable for the human brain, and as a result, we can confidently say that it is enabling more robots to perform their task. Furthermore, it took only 100 ms to get the high-quality data which ensures a higher ROI.
Simply put, “See More, Do More.” If you can see more, you can do more.
Moving on, we have an example of metallic objects. This is an industrial bin filled with random metallic and shiny parts commonly used in the manufacturing and automotive industries.
The bin is captured using laser structured light technology. You can see the items, but some of them are missing data, making it harder to see the object’s shape, which is essential to localize a part. Some parts are just blurred, making it difficult to capture and pick the pieces with high efficiency.
Let’s compare the same scene with a high-end stereo camera. It took less time, 300 ms, but the 3D point cloud quality is poor compared to the example above. The data that was at least visible with the laser structured light camera is now merged into the bin. If you want to use this 3D information for pick and place applications, it would be very challenging to execute the operation smoothly and efficiently.
Both laser structured light and high-stereo cameras are the most commonly used 3D technologies in the industrial automation market. Compare those results with 3D point clouds from Zivid 2.
You not only get 3D point clouds with color information, but you also get the shape of the parts, enabling your robots to see and pick each part with high confidence. You can clearly see cylinders and flat metallic objects, which were hard to separate in the previous examples. For starters, it took only 300 ms to get this high-quality data. Besides, you also get Zivid’s ART (Artifacts Reduction Technology) to capture very reflective parts without any artifact errors.
We, at Zivid, are giving robots the confidence to see parts accurately. Seeing with confidence means that you can see the tiniest details with the highest precision. To see the parts with confidence, you need high-precision, high dynamic range (HDR), and more data with minimum occlusion and artifacts.
Our Zivid 3D cameras meet the fundamental requirements for vision-guided robotic applications through cutting-edge 3D technology. Learn more about the Zivid 3D cameras.
3. Pick with Confidence – Why trueness matters in 3D imaging?
Now let’s look at the picking stage of the application and see how Zivid cameras solve picking challenges for industrial and collaborative robots. Trueness is a critical concept to understand - it’s related to picking objects reliably. Trueness describes errors and how well the sensor is calibrated to reality. If you have a high trueness error, the result can be that the pick location is wrong - even if the data is precise.
Let me give you an example of a high trueness error. If you look at the picture below, this is an example of metallic parts randomly placed in a bin for pick and place purposes. The red parts show the point cloud on top of the actual objects in the bin for understanding. You can see that the point cloud doesn’t match the reality of objects in space.
This is known as trueness error, and it comes from scaling, rotation, and translation errors. If your robot is asked to pick one of the parts in the bin, it will see the red parts instead of the actual metallic objects and go to the wrong place to pick because the data is not true to reality.
You can solve this problem by lowering the trueness error. Then picking and gripping is much easier for the robot. With Zivid 2, you get the data as true to reality as possible, like the example below.
This time, your robot should be able to pick the correct item quickly. We call it “Pick with Confidence.” When you see objects with higher reliability, accuracy, and confidence, your robot can perform accurate picking. Read more about trueness in 3D imaging.
It is worth mentioning that our accuracy and precision performance will be consistent throughout the lifetime of our 3D cameras. Some vendors claim a high accuracy level at a particular temperature. But it does not guarantee the same performance in industrial environments due to temperature variations or durability issues over continuous operations and lifetime of the sensor. So it is important to consider that the vision system should be industrial grade enough to withstand these changing conditions and still provide accurate data. Read more about industrial-grade 3D.
4. On-arm versability – Unleashing the potential of on-arm mounting
The last requirement for pick and place applications is the versability and the choice of mounting. As automation is making its place industry-wide, we see many expansions in robotic applications, especially on-arm robot mounted scenarios for various applications. With Zivid 2, you have the option to mount the camera for a stationary setup. Or you can mount it on a robot arm for some applications which require a flexible field of view (FOV) or multi-view imaging from different angles for a complete 360-degree view to obtain a full 3D model of an object.
Additionally, the ultra-compact and fast Zivid 2 3D camera makes it possible to mount on a robot without impacting maneuverability, usable payload, and cycle time. Find out the best way to mount your 3D machine vision sensor.
5. Pick and place robotic applications – Real-world examples
We have picked four real-world examples of pick and place applications with Zivid cameras from different industries.
1) Sixpack – Food picker
Based in the Netherlands, SIXPAK is an experienced supplier of food packaging solutions and provides a flexible assembly of ready meals, meal components, salads and convenience food. SHEFF Foodpicker, the newest robot offering by SIXPAK enables faster and more accurate food handling to lower costs. The Zivid 1+ 3D camera is used for SHEFF Foodpicker to reliably detect, select, classify, and pick food components in a short time.
2) Robsen – Machine tending
Robsen Robotics enabled a turn-key solution called iRoCube for machine tending applications using Zivid’s 3D vision camera. iRoCube covers a variety of materials to reduce the need for customization and shorten the production cycle.
3) DHL – Depalletizing
Through the robotisation of its warehouses, global logistics leader DHL is increasing both the efficiency of its e-commerce operations and the satisfaction of its employees at the same time. For a labour-intensive depalletizing, picking and fulfilment operation, DHL turned to system integrator Robomotive for a flexible solution to cope with a highly dynamic business environment. The Zivid One 3D color camera played a key role in this advanced robot cell design.
4) Vamag – Inspection
Vamag is a leading supplier of wheel alignment systems for overhaul centers, tire repairers, and car repair shops in 46 countries. With over 40 years of experience in the automotive market, it strives to find the easiest and fastest way to align wheels of the vehicles. Zivid 1+ 3D cameras are used for Vamag’s latest contactless wheel alignment demonstration, where they detect the tiniest of misalignment defects incredibly fast.
6. Live demo of a Zivid Two 3D camera
You can watch the live demo of the Zivid 2 3D camera in the video.
If you need a personalized 3D demo, you can send us a request to this page:
https://www.zivid.com/schedule-a-free-zivid-demo
7. Q&As
The full webinar includes 40 minutes of my presentation and live demo, followed by 10 minutes Q&As from the audience. There were lots of interesting questions from the attendees. If you want to watch the full webinar, please click the link below.
If you have any questions about the webinar, please leave a comment or reach out to me via email or Linkedin.
You May Also Like
These Related Stories
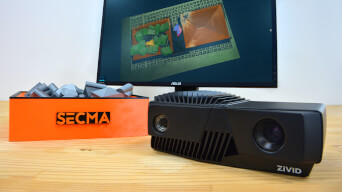
SECMA improves bin-picking with 3D vision
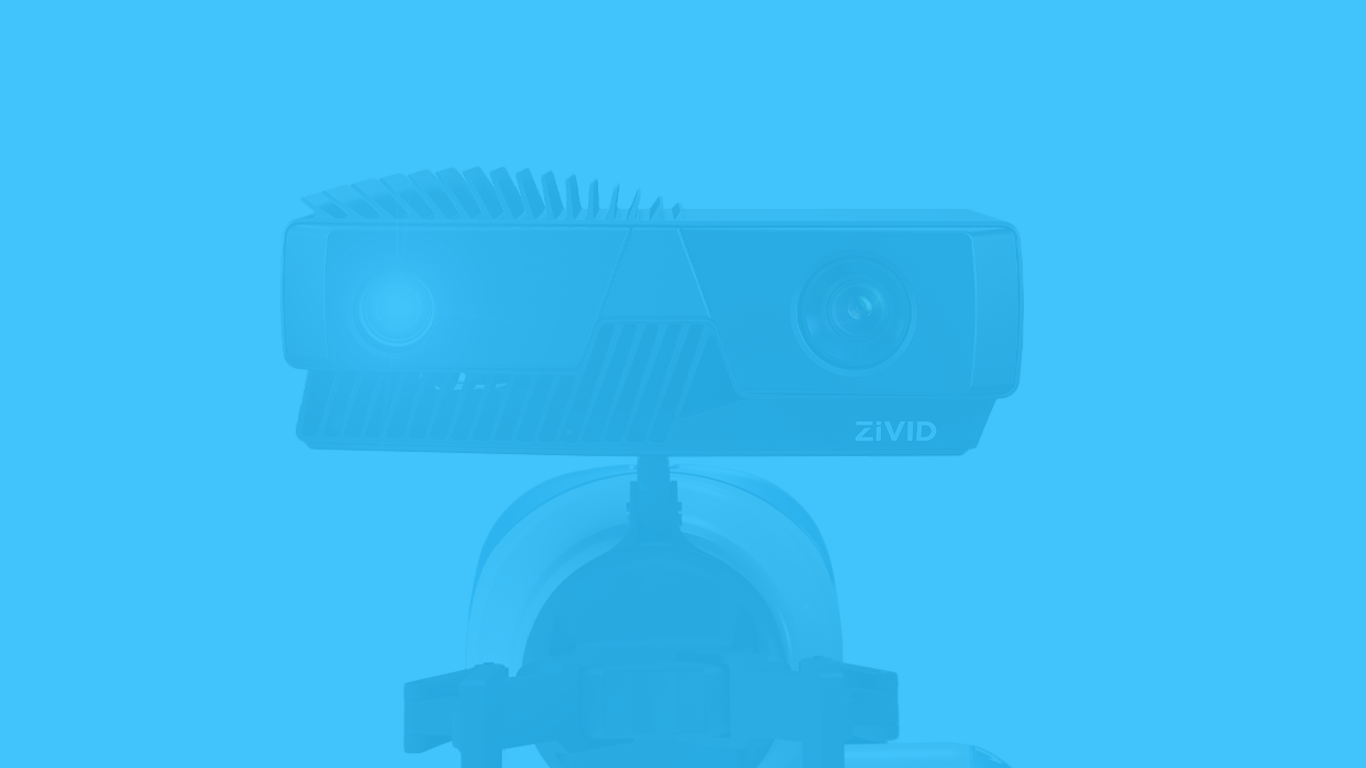
A beginner's guide to 3D machine vision cameras
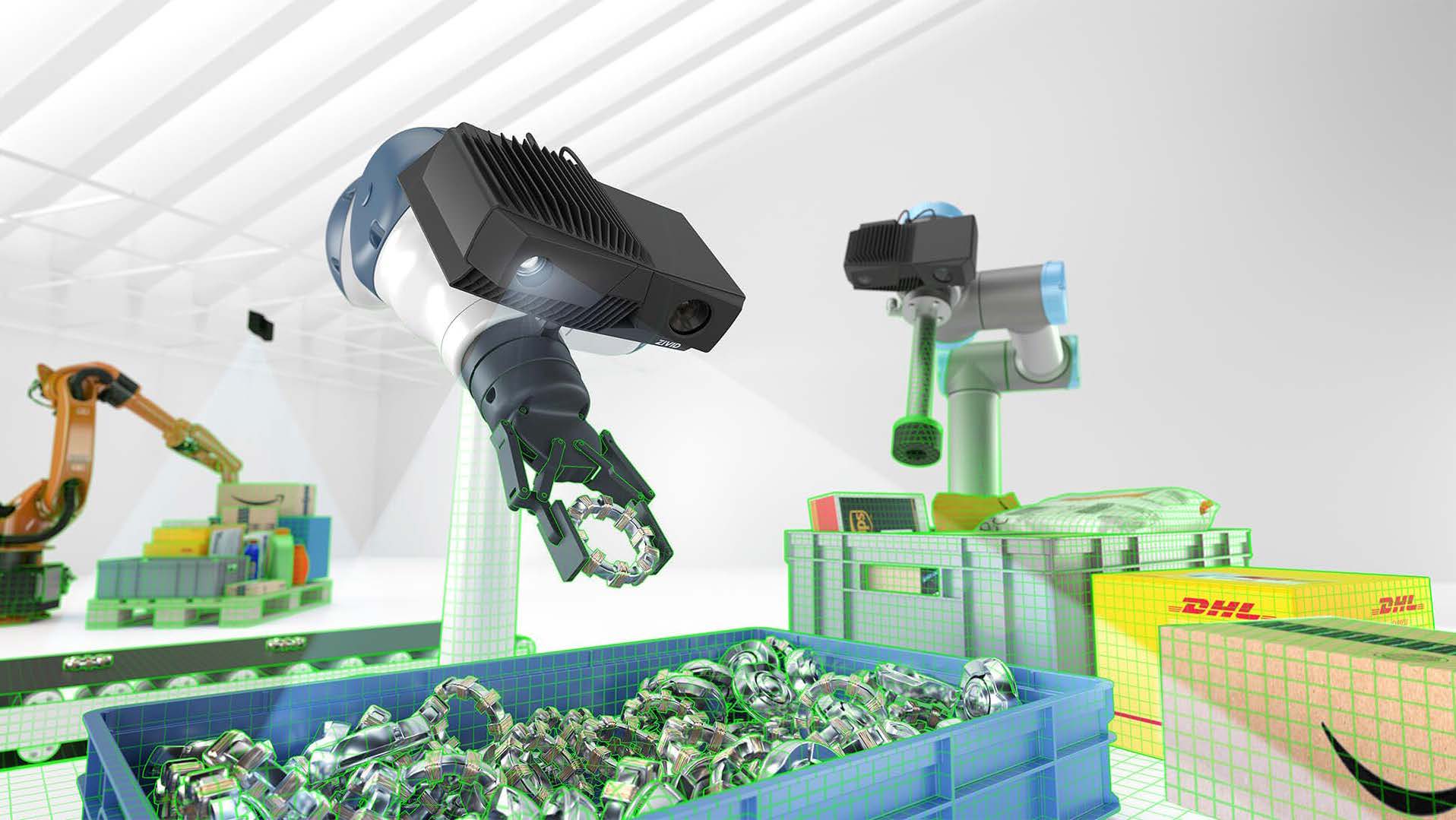